函数调用 API
在 AI 模型中集成函数支持,允许模型请求执行客户端函数,从而根据需要访问必要的信息或动态执行任务。
Spring AI 目前支持以下 AI 模型的函数调用:
-
Anthropic Claude:请参阅 Anthropic Claude 函数调用文档。
-
Azure OpenAI:请参阅 Azure OpenAI 函数调用文档。
-
Google VertexAI Gemini:请参阅 Vertex AI Gemini 函数调用文档。
-
Groq:请参阅 Groq 函数调用文档。
-
Mistral AI:请参阅 Mistral AI 函数调用文档。
-
Ollama:请参阅 Ollama 函数调用文档(尚不支持流式处理)。
-
OpenAI:请参阅 OpenAI 函数调用文档。
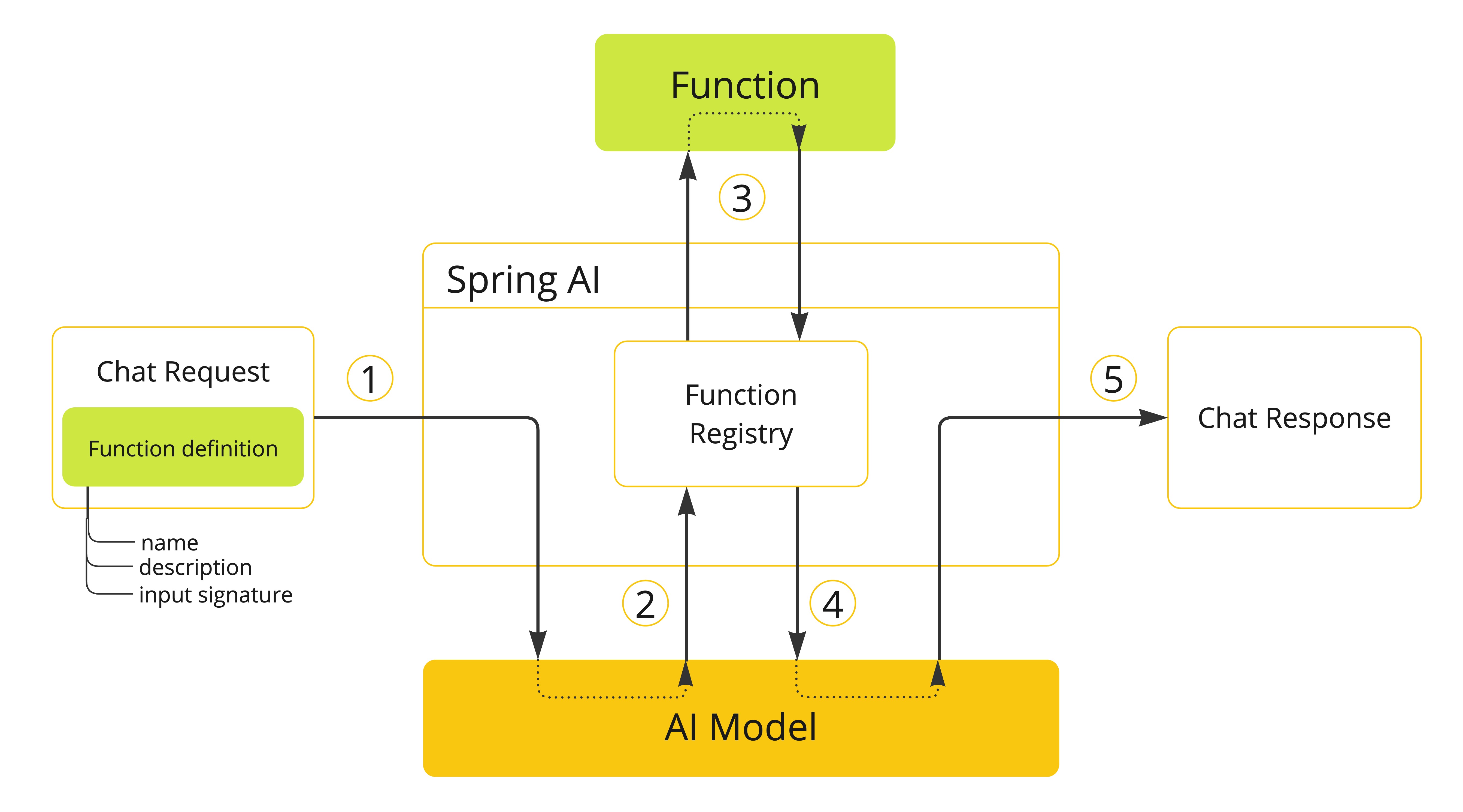
您可以使用ChatClient
并让 AI 模型智能地选择输出包含参数的 JSON 对象,以调用一个或多个已注册的函数。
这允许您将 LLM 功能与外部工具和 API 连接起来。
AI 模型经过训练,可以检测何时应该调用函数,并使用符合函数签名的 JSON 进行响应。
API 不直接调用该函数;相反,该模型会生成 JSON,您可以使用该 JSON 在代码中调用函数,并将结果返回给模型以完成对话。
Spring AI 提供了灵活且用户友好的方法来注册和调用自定义函数。
通常,自定义函数需要提供一个函数name
,description
和函数调用signature
(作为 JSON 架构)让模型知道函数需要哪些参数。这description
帮助模型了解何时调用函数。
作为开发人员,您需要实现一个函数,该函数采用从 AI 模型发送的函数调用参数,并将结果返回给模型。您的函数可以反过来调用其他第三方服务来提供结果。
Spring AI 使这就像定义@Bean
定义,该定义返回一个java.util.Function
并在调用ChatClient
或在提示请求中动态注册函数。
在后台, Spring 使用适当的适配器代码包装您的 POJO(函数),以便与 AI 模型进行交互,从而避免编写繁琐的样板代码。 底层基础架构的基础是 FunctionCallback.java 接口和配套的 Builder 实用程序类,以简化 Java 回调函数的实现和注册。
运作方式
假设我们希望 AI 模型使用它没有的信息进行响应,例如,给定位置的当前温度。
我们可以为 AI 模型提供有关我们自己的函数的元数据,它可以在处理您的提示时使用这些元数据来检索该信息。
例如,如果在处理提示期间,AI 模型确定它需要有关给定位置温度的其他信息,它将启动服务器端生成的请求/响应交互。 AI 模型不会返回最终响应消息,而是在特殊的 Tool Call 请求中返回,提供函数名称和参数(作为 JSON)。 客户端负责处理此消息并执行命名函数并返回响应 作为 Tool Response 消息返回给 AI 模型。
Spring AI 大大简化了您需要编写以支持函数调用的代码。
它为您代理函数调用对话。
您可以简单地将函数定义作为@Bean
,然后在提示选项中提供函数的 Bean 名称,或在提示请求选项中直接将函数作为参数传递。
您还可以在提示符中引用多个函数 Bean 名称。
示例用例
让我们定义一个简单的用例,我们可以将其用作示例来解释函数调用的工作原理。 让我们创建一个聊天机器人,通过调用我们自己的函数来回答问题。 为了支持聊天机器人的响应,我们将注册自己的函数,该函数获取一个位置并返回该位置的当前天气。
当模型需要回答诸如"What’s the weather like in Boston?"
AI 模型将调用客户端,提供 location 值作为要传递给函数的参数。这种类似 RPC 的数据以 JSON 形式传递。
我们的函数调用一些基于 SaaS 的天气服务 API,并将天气响应返回给模型以完成对话。在此示例中,我们将使用一个名为MockWeatherService
对不同位置的温度进行硬编码。
以下内容MockWeatherService
class 表示天气服务 API:
-
Java
-
Kotlin
public class MockWeatherService implements Function<Request, Response> {
public enum Unit { C, F }
public record Request(String location, Unit unit) {}
public record Response(double temp, Unit unit) {}
public Response apply(Request request) {
return new Response(30.0, Unit.C);
}
}
class MockWeatherService : Function1<Request, Response> {
override fun invoke(request: Request) = Response(30.0, Unit.C)
}
enum class Unit { C, F }
data class Request(val location: String, val unit: Unit) {}
data class Response(val temp: Double, val unit: Unit) {}
服务器端注册
函数作为 Bean
Spring AI 提供了多种在 Spring 上下文中将自定义函数注册为 bean 的方法。
我们首先介绍对 POJO 最友好的选项。
普通函数
在此方法中,您将定义一个@Bean
就像你对待任何其他 Spring 托管对象一样。
在内部,Spring AIChatModel
将创建一个FunctionCallback
这增加了通过 AI 模型调用它的逻辑。
的名称@Bean
是使用函数名称。
-
Java
-
Kotlin
@Configuration
static class Config {
@Bean
@Description("Get the weather in location") // function description
public Function<MockWeatherService.Request, MockWeatherService.Response> currentWeather() {
return new MockWeatherService();
}
}
@Configuration
class Config {
@Bean
@Description("Get the weather in location") // function description
fun currentWeather(): (Request) -> Response = MockWeatherService()
}
这@Description
annotation 是可选的,它提供了一个函数描述,可帮助模型了解何时调用该函数。这是一个重要的属性,可帮助 AI 模型确定要调用的客户端函数。
提供函数描述的另一种方法是使用@JsonClassDescription
注解MockWeatherService.Request
:
-
Java
-
Kotlin
@Configuration
static class Config {
@Bean
public Function<Request, Response> currentWeather() { // bean name as function name
return new MockWeatherService();
}
}
@JsonClassDescription("Get the weather in location") // function description
public record Request(String location, Unit unit) {}
@Configuration
class Config {
@Bean
fun currentWeather(): (Request) -> Response { // bean name as function name
return MockWeatherService()
}
}
@JsonClassDescription("Get the weather in location") // function description
data class Request(val location: String, val unit: Unit)
最佳实践是使用信息对请求对象进行注释,以便为该函数生成的 JSON 架构尽可能具有描述性,以帮助 AI 模型选择要调用的正确函数。
函数回调
注册函数的另一种方法是创建一个FunctionCallback
喜欢这个:
-
Java
-
Kotlin
@Configuration
static class Config {
@Bean
public FunctionCallback weatherFunctionInfo() {
return FunctionCallback.builder()
.function("CurrentWeather", new MockWeatherService()) // (1) function name and instance
.description("Get the weather in location") // (2) function description
.inputType(MockWeatherService.Request.class) // (3) input type to build the JSON schema
.build();
}
}
import org.springframework.ai.model.function.withInputType
@Configuration
class Config {
@Bean
fun weatherFunctionInfo(): FunctionCallback {
return FunctionCallback.builder()
.function("CurrentWeather", MockWeatherService()) // (1) function name and instance
.description("Get the weather in location") // (2) function description
// (3) Required due to Kotlin SAM conversion being an opaque lambda
.inputType<MockWeatherService.Request>()
.build();
}
}
它包装了第三方MockWeatherService
函数并将其注册为CurrentWeather
函数替换为ChatClient
.
它还提供了一个描述 (2) 和一个可选的响应转换器,用于将响应转换为模型预期的文本。
默认情况下,响应转换器执行 Response 对象的 JSON 序列化。 |
这FunctionCallback.Builder 内部根据MockWeatherService.Request 类。 |
按 Bean 名称启用函数
要让模型知道并调用您的CurrentWeather
函数,以便在提示请求中启用它:
ChatClient chatClient = ...
ChatResponse response = this.chatClient.prompt("What's the weather like in San Francisco, Tokyo, and Paris?")
.functions("CurrentWeather") // Enable the function
.call().
chatResponse();
logger.info("Response: {}", response);
上述用户问题将触发对CurrentWeather
函数(每个城市一个),最终响应将如下所示:
Here is the current weather for the requested cities: - San Francisco, CA: 30.0°C - Tokyo, Japan: 10.0°C - Paris, France: 15.0°C
客户端注册
除了自动配置之外,您还可以动态注册回调函数。
您可以使用函数调用或方法调用方法将函数注册到ChatClient
或ChatModel
请求。
默认情况下,客户端注册使您能够注册函数。
函数调用
ChatClient chatClient = ...
ChatResponse response = this.chatClient.prompt("What's the weather like in San Francisco, Tokyo, and Paris?")
.functions(FunctionCallback.builder()
.function("currentWeather", (Request request) -> new Response(30.0, Unit.C)) // (1) function name and instance
.description("Get the weather in location") // (2) function description
.inputType(MockWeatherService.Request.class) // (3) input type to build the JSON schema
.build())
.call()
.chatResponse();
默认情况下,在此请求的持续时间内,动态功能处于启用状态。 |
这种方法允许根据用户输入动态选择要调用的不同函数。
FunctionCallbackInPromptIT.java 集成测试提供了一个完整的示例,说明如何使用ChatClient
并在提示请求中使用它。
方法调用
这MethodInvokingFunctionCallback
支持通过反射进行方法调用,同时自动处理 JSON 架构生成和参数转换。
它对于将 Java 方法作为可调用函数集成到 AI 模型交互中特别有用。
这MethodInvokingFunctionCallback
实现FunctionCallback
接口并提供:
-
为方法参数自动生成 JSON 架构
-
支持 static 和 instance 方法
-
任意数量的参数(包括 none)和返回值(包括 void)
-
任何参数/返回类型(基元、对象、集合)
-
特殊处理
ToolContext
参数
您需要FunctionCallback.Builder
创建MethodInvokingFunctionCallback
喜欢这个:
// Create using builder pattern
FunctionCallback methodInvokingCallback = FunctionCallback.builder()
.method("MethodName", Class<?>...argumentTypes) // The method to invoke and its argument types
.description("Function calling description") // Hints the AI to know when to call this method
.targetObject(targetObject) // Required instance methods for static methods use targetClass
.build();
以下是一些使用示例:
-
Static Method Invocation
-
Instance Method with ToolContext
public class WeatherService {
public static String getWeather(String city, TemperatureUnit unit) {
return "Temperature in " + city + ": 20" + unit;
}
}
// Usage
FunctionCallback callback = FunctionCallback.builder()
.method("getWeather", String.class, TemperatureUnit.class)
.description("Get weather information for a city")
.targetClass(WeatherService.class)
.build();
public class DeviceController {
public void setDeviceState(String deviceId, boolean state, ToolContext context) {
Map<String, Object> contextData = context.getContext();
// Implementation using context data
}
}
// Usage
DeviceController controller = new DeviceController();
String response = ChatClient.create(chatModel).prompt()
.user("Turn on the living room lights")
.functions(FunctionCallback.builder()
.method("setDeviceState", String.class,boolean.class,ToolContext.class)
.description("Control device state")
.targetObject(controller)
.build())
.toolContext(Map.of("location", "home"))
.call()
.content();
OpenAiChatClientMethodInvokingFunctionCallbackIT 集成测试提供了如何使用 FunctionCallback.Builder 创建方法调用 FunctionCallbacks 的额外示例。
工具上下文
Spring AI 现在支持通过工具上下文将额外的上下文信息传递给函数回调。 此功能允许您提供额外的用户提供的数据,这些数据可与 AI 模型传递的函数参数一起在函数执行中使用。
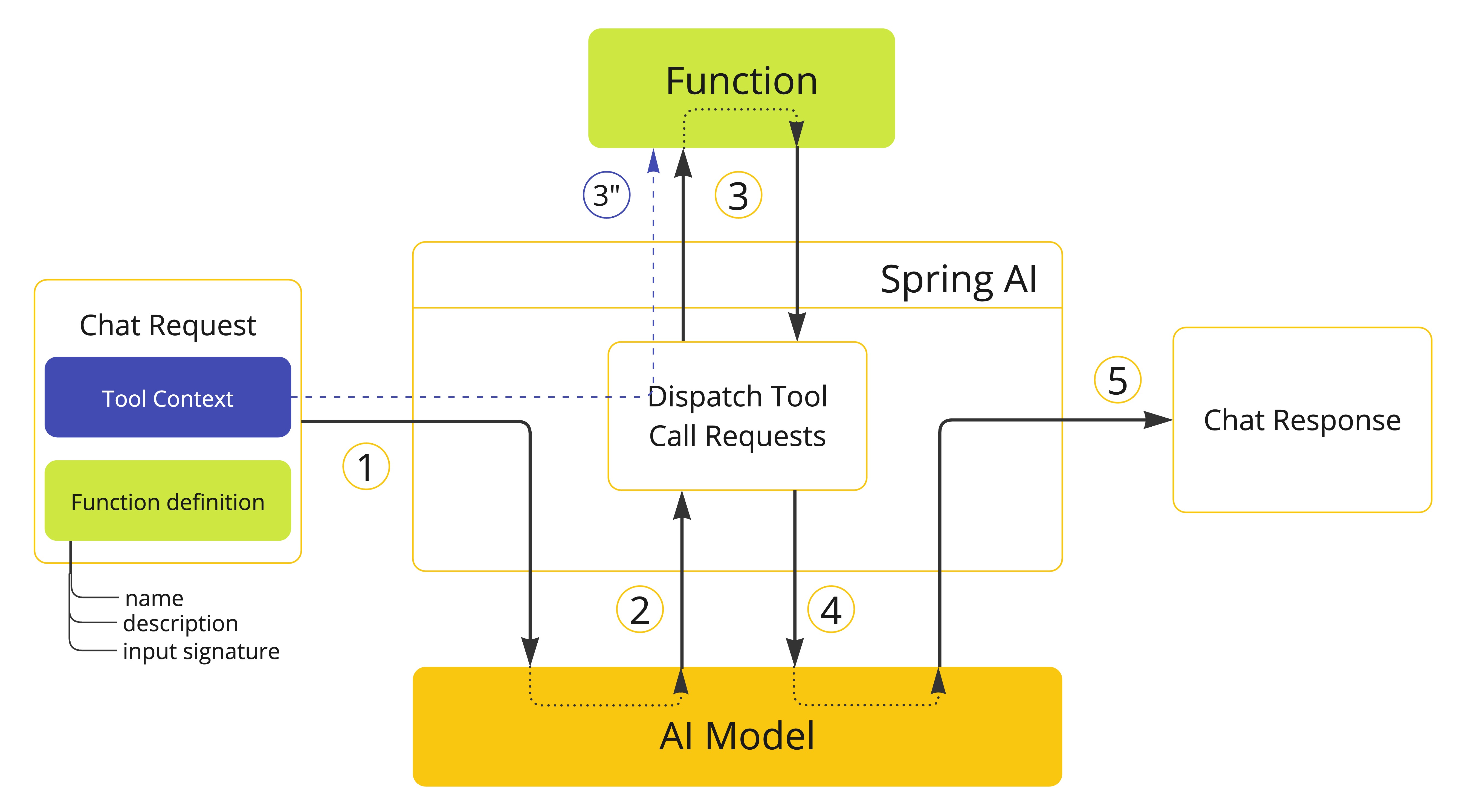
ToolContext 类提供了一种传递其他上下文信息的方法。
使用工具上下文
在函数调用的情况下,作为java.util.BiFunction
.
对于方法调用,上下文信息作为 type 为ToolContext
.
函数调用
您可以在构建聊天选项时设置工具上下文,并将 BiFunction 用于回调:
BiFunction<MockWeatherService.Request, ToolContext, MockWeatherService.Response> weatherFunction =
(request, toolContext) -> {
String sessionId = (String) toolContext.getContext().get("sessionId");
String userId = (String) toolContext.getContext().get("userId");
// Use sessionId and userId in your function logic
double temperature = 0;
if (request.location().contains("Paris")) {
temperature = 15;
}
else if (request.location().contains("Tokyo")) {
temperature = 10;
}
else if (request.location().contains("San Francisco")) {
temperature = 30;
}
return new MockWeatherService.Response(temperature, 15, 20, 2, 53, 45, MockWeatherService.Unit.C);
};
ChatResponse response = chatClient.prompt("What's the weather like in San Francisco, Tokyo, and Paris?")
.functions(FunctionCallback.builder()
.function("getCurrentWeather", this.weatherFunction)
.description("Get the weather in location")
.inputType(MockWeatherService.Request.class)
.build())
.toolContext(Map.of("sessionId", "1234", "userId", "5678"))
.call()
.chatResponse();
在此示例中,weatherFunction
定义为将请求和工具上下文作为参数的 BiFunction。这允许您直接在函数 logic中访问上下文。
这种方法允许您将特定于会话或用户的信息传递给您的函数,从而实现更多上下文和个性化的响应。
方法调用
public class DeviceController {
public void setDeviceState(String deviceId, boolean state, ToolContext context) {
Map<String, Object> contextData = context.getContext();
// Implementation using context data
}
}
// Usage
DeviceController controller = new DeviceController();
String response = ChatClient.create(chatModel).prompt()
.user("Turn on the living room lights")
.functions(FunctionCallback.builder()
.method("setDeviceState", String.class,boolean.class,ToolContext.class)
.description("Control device state")
.targetObject(controller)
.build())
.toolContext(Map.of("location", "home"))
.call()
.content();